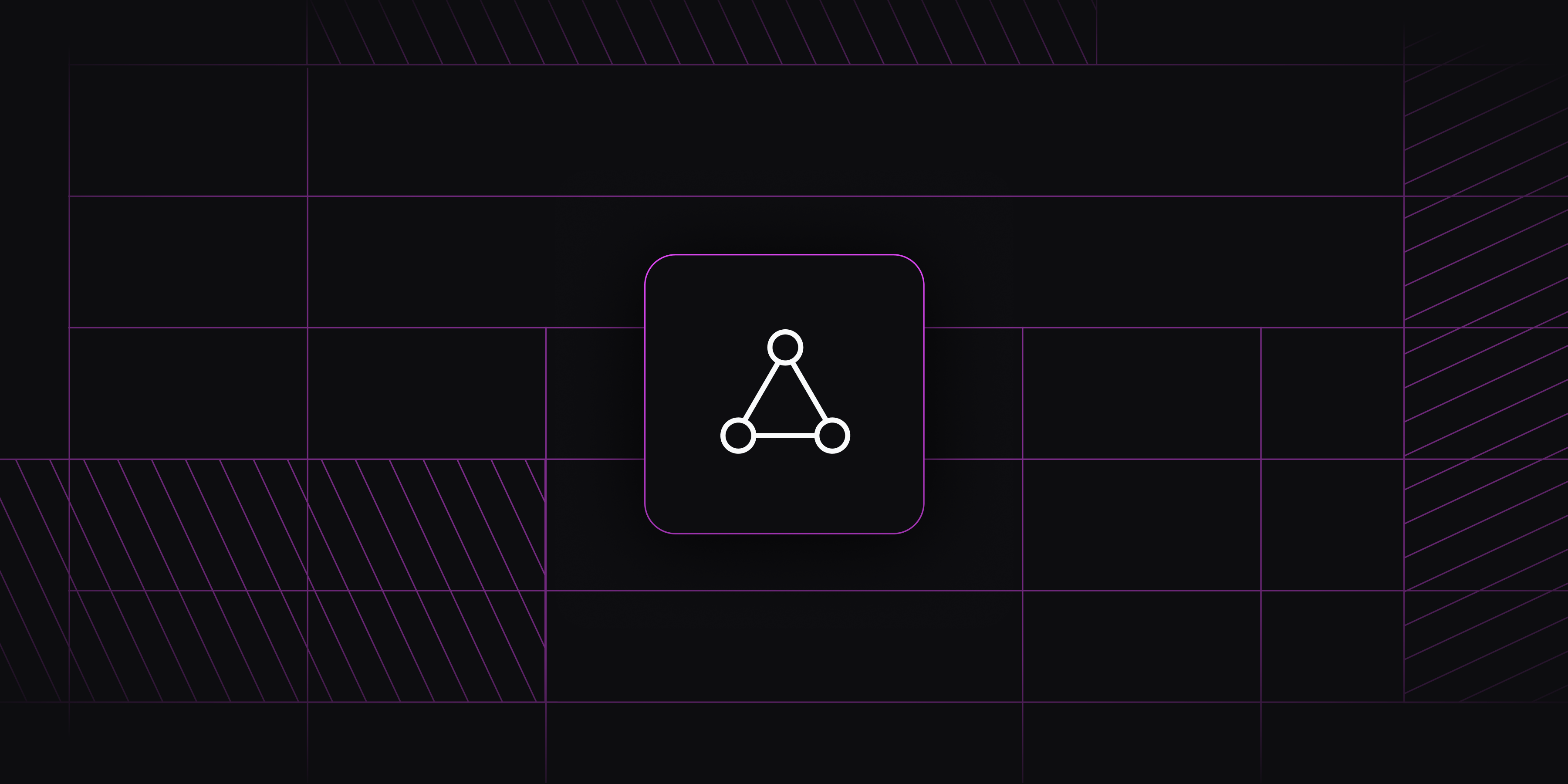
Common RAG Problems: AI Data Segmentation
Retrieval Augmented Generation (RAG) systems are powerful, but effectively managing and retrieving the right data from vast knowledge bases can present challenges. One common problem revolves around organizing and segmenting your data.
When deciding if you should segment your AI data you may ask yourself a few key questions depending on your use case:
- Do I need to ensure users only access relevant information?
- Do I need to prevent data leakage in a multi-tenant application?
- Do I need to keep knowledge bases focused to improve retrieval accuracy?
If the answer to any of these questions is “Yes”, then you may consider segmenting your AI data when indexing it in a RAG system.
The need for AI data segmentation
In many RAG use cases, simply dumping all documents into a single pool isn't sufficient. Consider a multi-tenant SaaS application where each customer has their own private documents, or an organization with distinct knowledge bases for legal, HR, and customer support. Without proper segmentation, retrievals could accidentally pull data from the wrong tenant or domain, leading to privacy issues or irrelevant results.
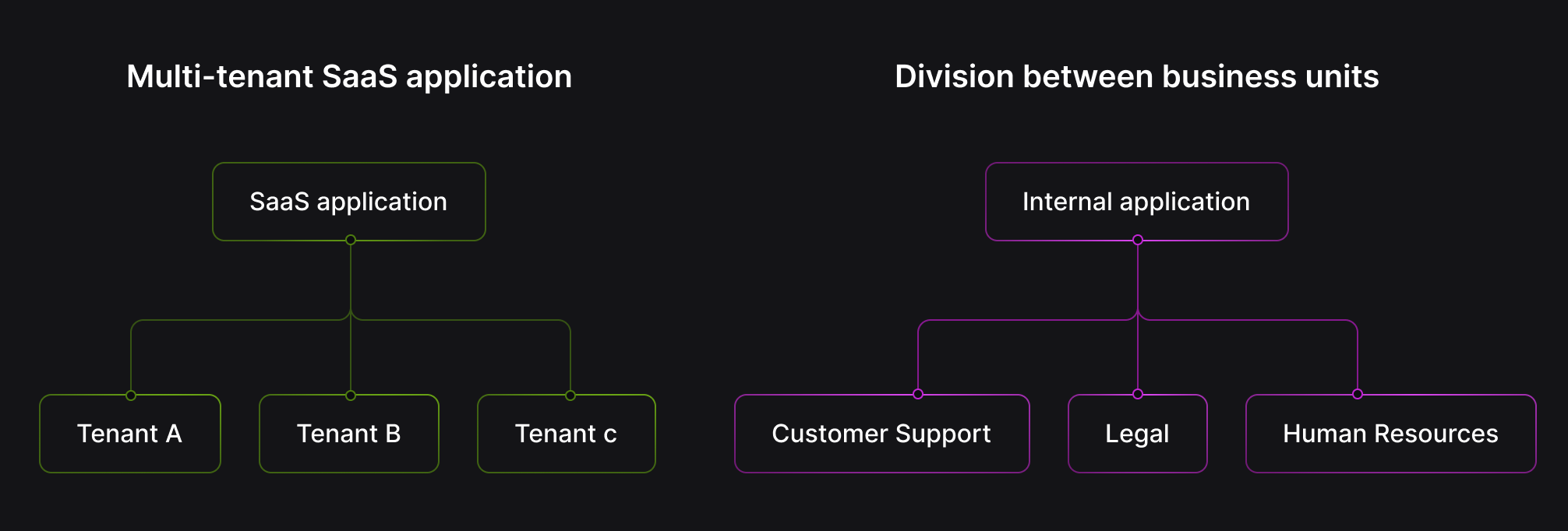
Benefits of AI data isolation
Using hard logical boundaries, or partitions, for data within Retrieval Augmented Generation systems offers significant advantages. Primarily, partitions enable data isolation, which is critical in applications handling data from multiple sources or users, such as multi-tenant environments, to prevent data leakage between distinct datasets. This ensures that a query only accesses the information it is authorized to see.
Beyond security, partitioned logical boundaries are vital for improving retrieval accuracy and relevance. By allowing retrievals to be scoped to partitions, the system can focus its search on the most relevant pool of information. This focus can enhance the quality of retrieval, particularly in hybrid search methods where metrics like keyword importance are more accurate when calculated within a well-defined, domain-specific collection of documents.
AI data segmentation to improve retrieval accuracy
While partitions can be useful for data isolation and preventing data leakage, they also serve a critical role in improving hybrid search retrieval results. During hybrid search retrieval, a query is made to a keyword index as well as a semantic index and both results are combined using an Reciprocal Rank Fusion (RRF) score.
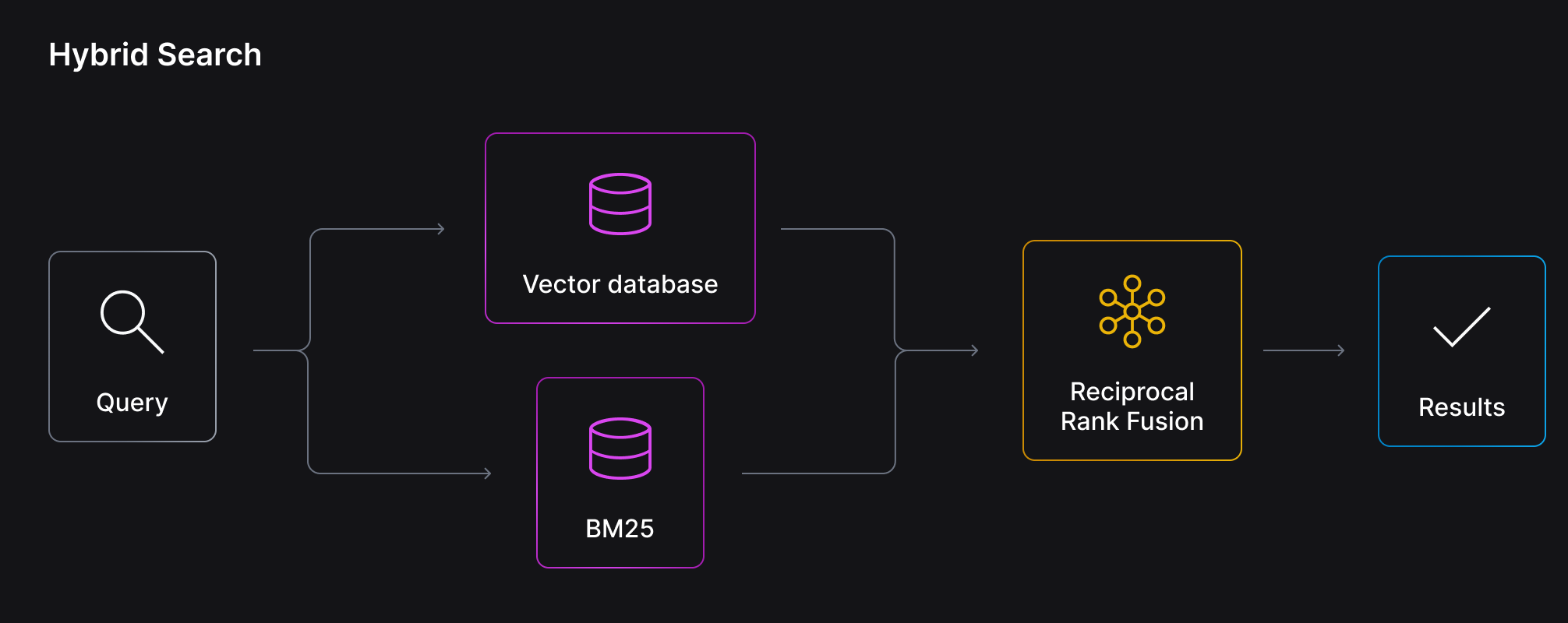
Data segmentation is particularly important in the keyword search leg of hybrid search retrieval. This is because keyword indexes use a method of scoring called TF-IDF where keyword importance (or weight) is partially determined by how frequently a keyword appears in the set of documents relative to its inverse document frequency.
For example, legal jargon in a set of law documents would be considered relatively less important compared to the same legal jargon appearing in customer service documents, since presumably the legal terms would appear far more frequently in the law documents. By segmenting documents into partitions based on domain, the quality of the keyword portion of a hybrid search approach can be improved because the relative importance of keywords is calculated within the context of that specific partition.
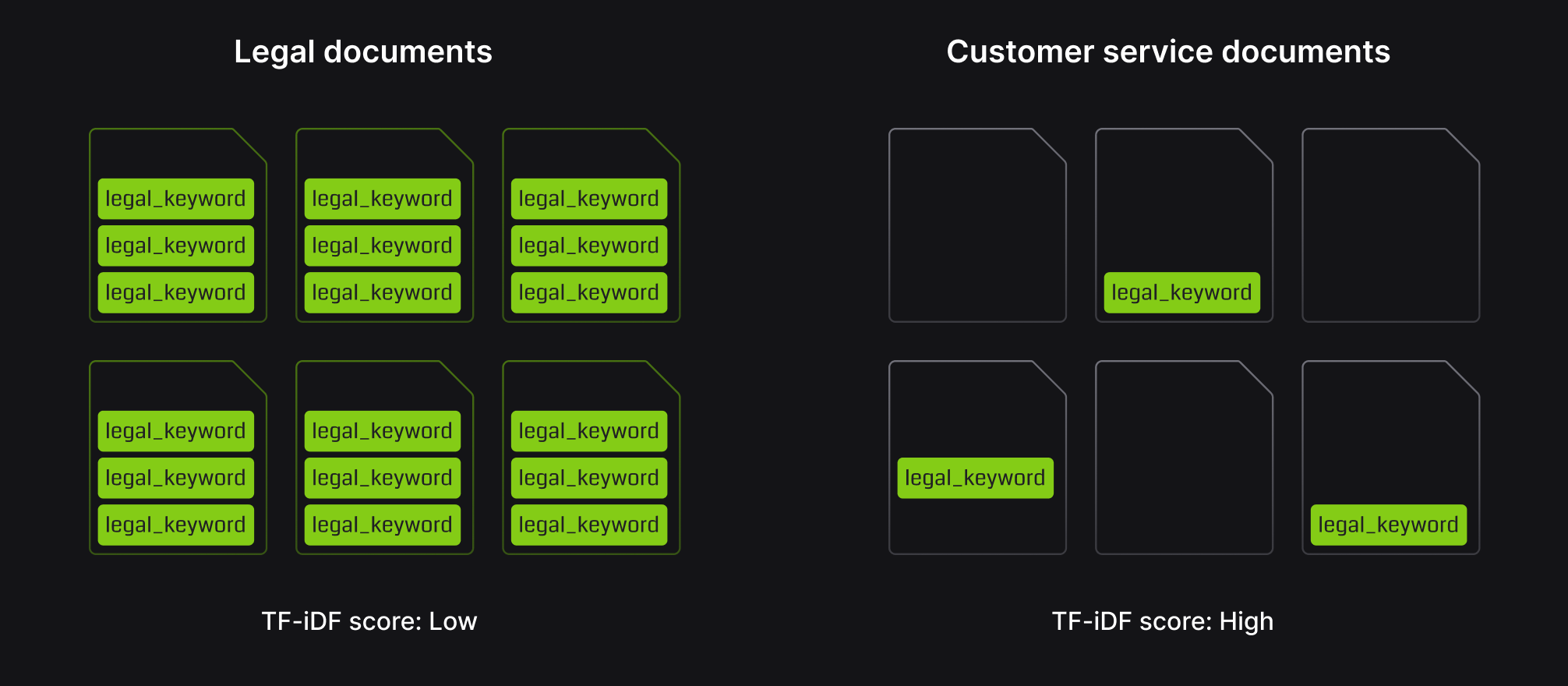
Partitions are equally as important in a multi-tenant application context where different tenants may have wildly different corpuses and neglecting to separate keyword indexes could lead to low quality search results for all tenants.
AI data segmentation with metadata and retrieval filters
While partitions provide high-level isolation for user data or separate knowledge bases, metadata filtering enables even more precise retrieval within these boundaries.
In a robust system, each document can be tagged with metadata properties such as document type, source, user_id, and/or additional customer key-value pairs. On retrieval, results can be filtered by these key-value pairs.
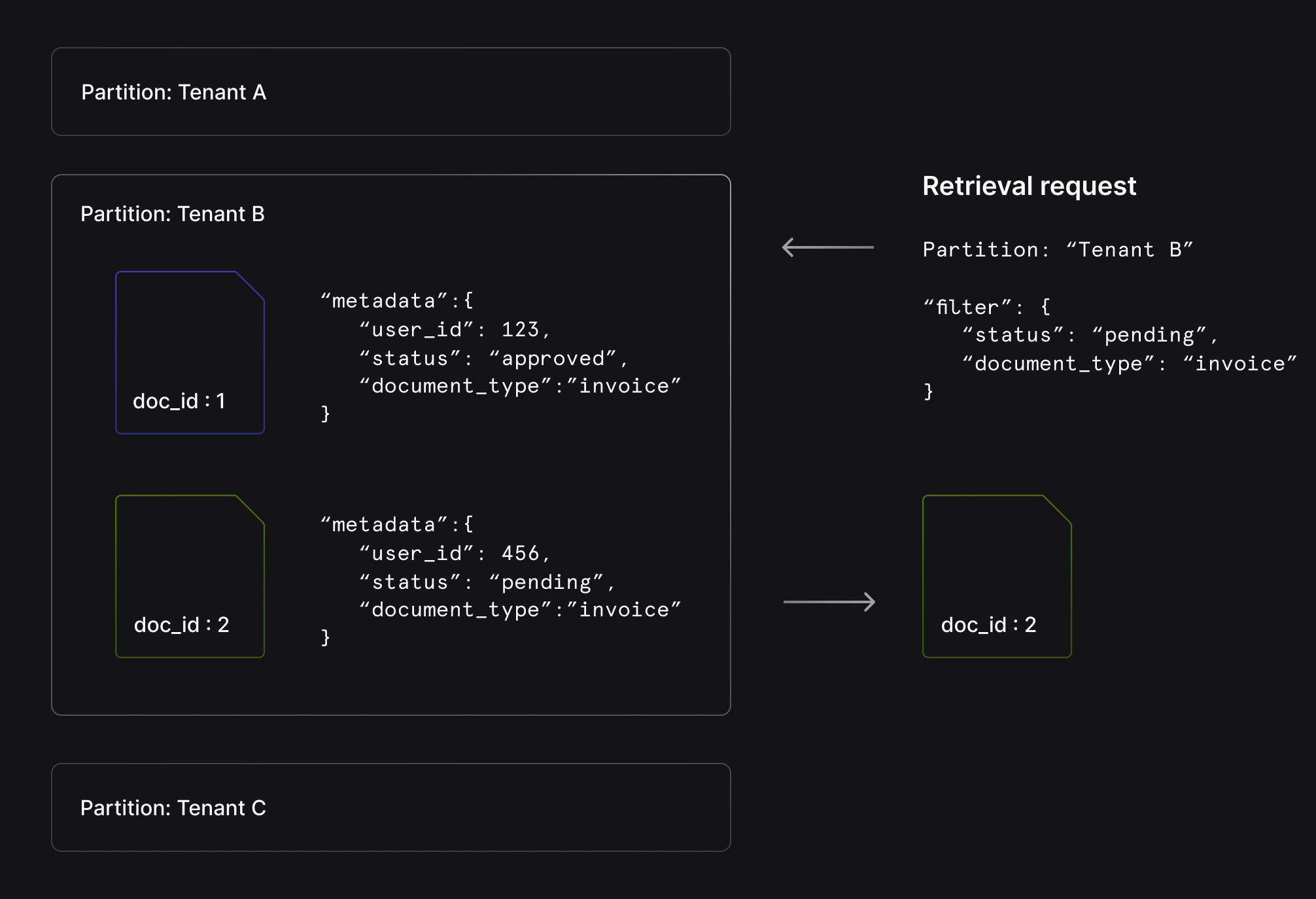
By combining partition scoping with metadata filters, you achieve fine-grained retrieval control that guarantees the retrieval of top results matching specific criteria. This powerful combination is particularly valuable in multi-tenant applications, where partitions first isolate organizational data, and metadata filters then target specific document attributes within that isolated context.
And although metadata filtering doesn’t typically represent a hard logical separation of data, metadata filtering can be used to further restrict data access in applications where it can even be used when implementing Role Based Access Controls (RBAC).
Final thoughts on AI data segmentation
Effective AI data segmentation is critical for RAG systems, delivering three core benefits:
1. Security: Partitions create logical boundaries that prevent data leakage across tenants or domains.
2. Retrieval quality: Domain-specific partitioning improves both semantic and keyword search, with properly contextualized TF-IDF calculations enhancing result relevance.
3. Precision control: Metadata filtering within partitions enables targeted retrievals based on document attributes, supporting fine-grained access controls when needed.
Whether building multi-tenant applications or domain-specific knowledge bases, implementing appropriate segmentation should be considered essential infrastructure rather than an optional feature—it fundamentally enhances the security, accuracy, and performance of your entire RAG system.
Using Ragie for AI data segmentation
Ragie has out of the box support for AI data segmentation using its partitions and metadata filtering. Below is an example of just how easy it is to quickly reap the benefits of data isolation, relevancy and fine grained control in just a few API calls.
# Set your API key as an environment variable
# Get a free API key by signing up at https://secure.ragie.ai
export RAGIE_API_KEY="your_api_key_here"
# 1. Create a document in the "legal" partition with metadata
curl --request POST \
--url https://api.ragie.ai/documents/raw \
--header "accept: application/json" \
--header "authorization: Bearer $RAGIE_API_KEY" \
--header "content-type: application/json" \
--data '{
"metadata": {"type": "contract"},
"partition": "legal",
"data": "Very important legal jargon content here."
}'
# 2. Create a document in the "hr" partition with different metadata
curl --request POST \
--url https://api.ragie.ai/documents/raw \
--header "accept: application/json" \
--header "authorization: Bearer $RAGIE_API_KEY" \
--header "content-type: application/json" \
--data '{
"metadata": {"type": "policy", "year": 2025},
"partition": "hr",
"data": "Employee handbook policy here."
}'
# 3. Retrieve documents from just the "legal" partition
curl https://api.ragie.ai/retrievals \
-X POST \
-H "Authorization: Bearer $RAGIE_API_KEY" \
-H "Content-Type: application/json" \
--data '{
"query": "What are the contract terms?",
"partition": "legal",
"top_k": 5
}'
# 4. Retrieve documents from the "hr" partition with metadata filtering
curl https://api.ragie.ai/retrievals \
-X POST \
-H "Authorization: Bearer $RAGIE_API_KEY" \
-H "Content-Type: application/json" \
--data '{
"query": "employee policies",
"partition": "hr",
"filter": {
"type": "policy",
"year": {"$gte": 2020}
},
"top_k": 3
}'
In this example, we created documents in separate "legal" and "hr" partitions with metadata, and then demonstrated targeted retrievals using partition scoping and metadata filtering.
You can try this yourself by signing up for free at secure.ragie.ai today.
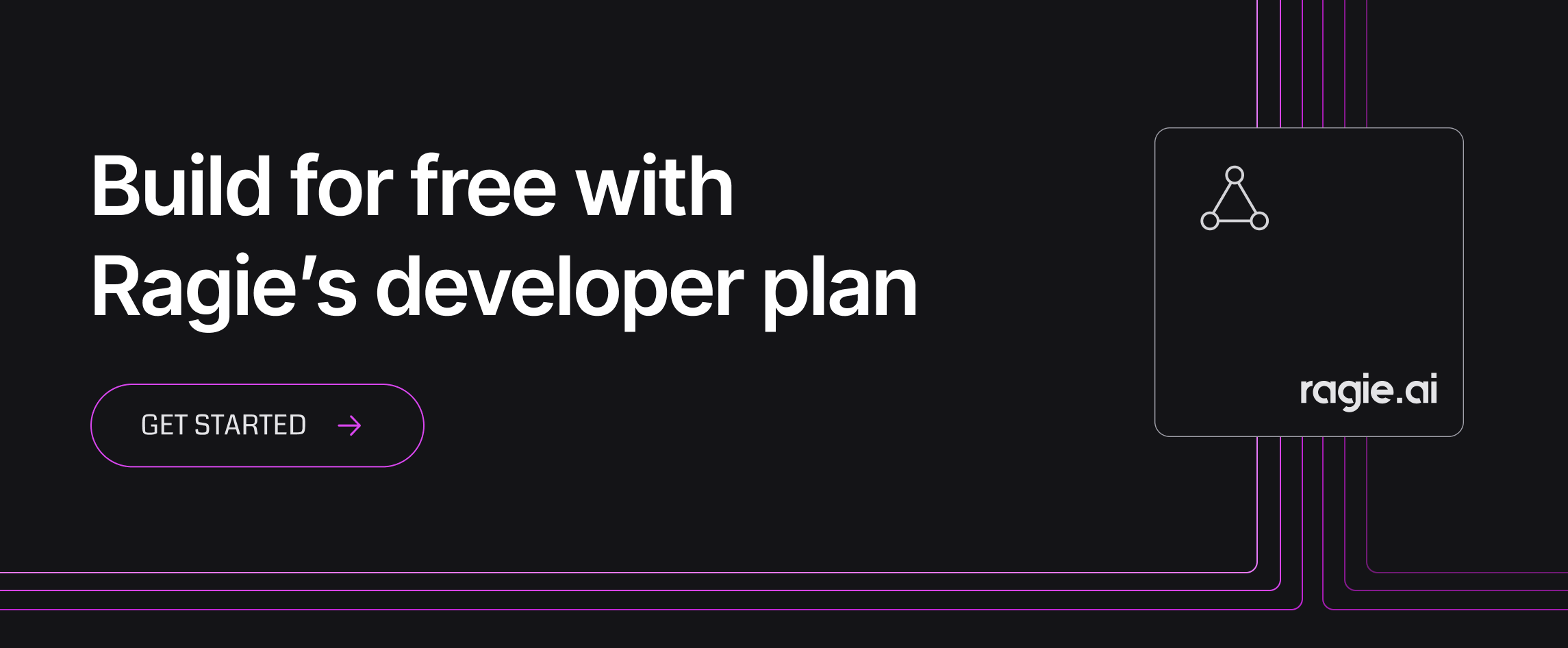